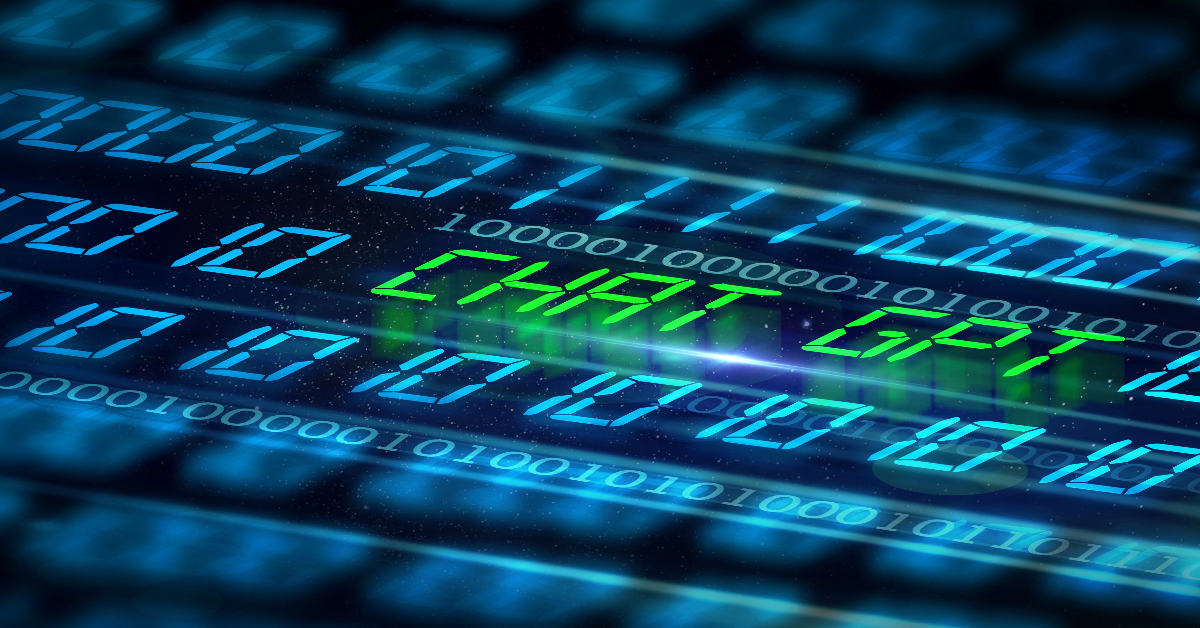
Our approach to the use of Artificial Intelligence (AI) is very much in line with the other services available through our platform - we already offer more conventional modules that can map, transform and parse data and the use of Large Language Models (LLM) is a fantastic extension of these for use in the right circumstances. The answer as to its use is not an either/or - with ipushpull you can use a combination of approaches within the same workflow. For example, different stages of a process may use different mapping tools or each step might use a combination to see which result is best - either sequentially or simultaneously.
We like to solve problems in a practical manner – we want to see how technology helps the day-to-day, minute-to-minute tasks that our customers must perform to ensure their business operates as it should. Amongst other things technology should improve efficiency and therefore lower costs, it should generate revenue through offering more opportunities and it should reduce risk by removing operationally risky manual processes.
When we look at AI we tend to come back to the above metrics to help understand where the value is right now to our customers. AI is of course rapidly evolving as it may offer tools in the future that are currently not used or thought possible – but we have to start somewhere and this feels like a good time to assess the hype vs substance given where we lie on the “curve”. Gartner has produced a great infographic (below) on the AI hype cycle – you don’t even need to read it to guess where we might be.
ipushpull is a data sharing and workflow platform – we have a number of tools that allow users to maximise the value and utility of their data within the application of their choice. The value we add with AI is twofold – the knowledge of where best to use it and the ability to integrate it into your business.
The focus here is on the former but the latter is also covered in our other blogs and through our mantra of delivering the right data, at the right time, in the right place.
We’ve undertaken a number of projects looking at refining processes and workflows through the use of LLM. It’s been easy to integrate these into the rest of the platform and hence we’ve been able to show front-to-back workflows where AI plays a significant role. We used multiple models across multiple providers to interpret chat messages and construct data objects for booking or pricing purposes, to improve the natural language capabilities of bots and to standardise data across hundreds of csv files each containing the same type of data in a different layout and schema.
We found that it performed exceptionally well on some tasks and less well on others. We started mapping out a spectrum of available tools and techniques to gauge where AI could fit and came up with the below model. There should be an accompanying 3 (plus) dimensional chart of how performance, cost, flexibility, data usage, and infosec characteristics intersect but I can't get Midjourney or DALL·E 3 to plot it.
The main takeaway for us was that there are often multiple solutions and the most suitable one needs to be determined with reference to the above characteristics – even if it is not the most exciting or hyped approach. In one example we questioned how accurate the models needed to be – should you aim for 100% accuracy if the underlying data could be stale or not particularly trustworthy? Is each individual piece of data significant or is the overall pattern more valuable? This question is important if the data you are now collecting has never been recorded, quantified, structured or analysed before in an automated fashion.
In the work we looked at there was a clear trade-off between timeliness and flexibility - we strongly believe the models will be exponentially quicker in the near future and even if not the benefit of having a global, flexible tool to apply across several workflows may be significantly more valuable than losing a few milliseconds here and there.
When choosing an approach it is also worth considering as well as how "structured" the data already is and how fast you want to deliver - training a model can take significant time but a technique such as prompt engineering is much quicker and allows you to iterate and fail fast.
Prompt engineering is essentially supplying additional context, instructions and examples to a model to deal with your particular use case. It is quick to update but in contrast to other forms of "coding" it is not deterministic- changing one aspect of a prompt to correct an issue can lead to an unintended effect on the accuracy of previous results. Writing the prompts is therefore a skill in itself and one which we "failed fast" at to get to where we are now.
A final note on the use of LLM - you do not have to be restricted to using a large provider - there are open source models available which can be tailored to your own use case, reduce cost short and long term, provide a better fit and valuable retention of IP and assets. We can advise, implement and integrate with the rest of our platform.
While the applications of AI are many we’re also continually reminded, as we innovate with our customers and collaborators, that the power of AI is useless until it’s integrated into workflows in a usable way. ipushpull is uniquely positioned to deliver such integrated solutions and we’d love to explore how to maximise the benefits to you. Why not contact us to find out more?'